At the recent Square Peg 2023 AGM, investors Yonatan Sela and Casey Flint hosted a deep dive into AI, as the industry continues to develop at a breakneck pace. They shared their thoughts on this next ‘wave’ of technology shift, tailwinds for growth, and their predictions for what comes next.
AI: The Next Big ‘Wave’ In The Technology Shift
The major innovations and subsequent value capture within technology have centred around key ‘waves’, where each wave creates a brand new canvas of opportunities.
Companies like IBM and Intel led breakthroughs in the desktop computer and microprocessors that form the foundational hardware we use for most technologies today, while other companies like Microsoft capitalised on the software layer with their dominant Windows operating system so that we can easily use this hardware. We’ve also seen winners from previous waves create a lot of value in new waves. An example is Amazon using its significant scale from its success in ecommerce over the internet to build Amazon Web Services (AWS) in the Cloud wave.
The emergence of the AI wave is the same. There will be beneficiaries from previous waves accumulating immense value as well as entirely new entrants.

Back to basics: AI Terminology
Because of how much interest there is in AI currently, many people misunderstand the key terms. We’ve tried to simplify a few of these below:
- Artificial Intelligence (AI): a branch of computer science that seeks to develop technologies that bring human-level capabilities to machines, such that they can achieve tasks that usually require human-level intelligence.
- Machine Learning (ML): a subset of AI referring to the set of techniques to achieve AI (excluding some less prominent domains).
- Deep Learning (DL): a subset of ML, refers to constructing models with a “deep” (or large) number of layers in the form of a neural net.
- Generative AI (GenAI): a subset of DL, refers to models that generate new data points by statistically modelling and replicating the distribution of the data it was trained on.
- Artificial General Intelligence (AGI): While there’s no perfect consensus on the definition of AGI, it typically refers to an AI that can solve a wide variety of problems, as opposed to only being capable of solving one narrow or specialised problem.
What makes this wave different?
There are three main tailwinds driving the recent inflection point in AI: data proliferation, compute (FLOPS) growth, and research breakthroughs.
Data Proliferation
In the old world of AI, most models were data-constrained, limiting their development and overall effectiveness. However, since the technological wave of the Internet, we now have enormous amounts of data available online. While the creation of this data was initially centralised in Web 1.0, limited mainly to website owners, the shift to user-generated content within Web 2.0 has led to the huge proliferation of data and language that can be used for model training. This is in large part thanks to social media platforms like Facebook and Reddit enabling anyone on the web to create content. Today, thanks to this proliferation of open data, models like those that power ChatGPT are trained on such a large volume of content that it would take 2000 years for one person to read it all.

Compute Growth
Compute performance (measured in FLOPS: floating point operations per second) has historically been increasing in line with Moore’s Law. Moore’s Law states that the number of transistors you can fit on an integrated circuit doubles every year, and that has a corresponding impact on performance. Architecture improvements, energy efficiency, and parallel processing capabilities have also contributed to increased compute performance over time.
More powerful chips and faster compute mean we’re able to process more of the mathematical operations required to train and use these large models in less time (and iterate faster as a result), as well as hold larger datasets.
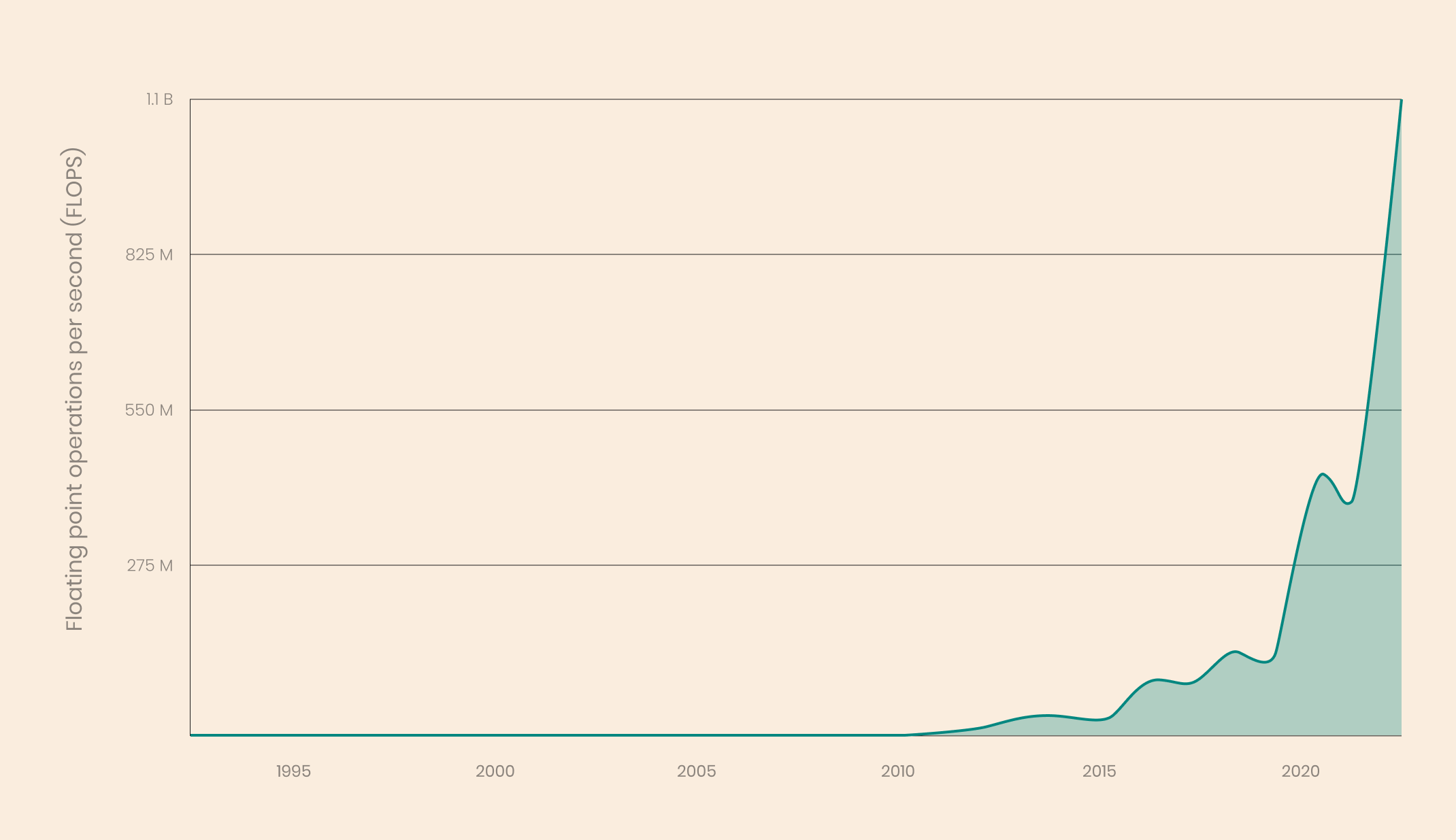
NB: While the changes stipulated to Moore’s Law have contributed greatly to compute increases, Moore’s Law is considered by many to no longer be effective.
Research Breakthroughs
While today people associate the term “generative AI” with the generation of content (text, images, code etc), when the term was first coined forty years ago it referred to models that could generate new data points that would resemble the underlying data the model was trained on.
In the last forty years, scientists have tried to make generative models perform well, but have struggled against its high need for computational power and data and the complexity of generative model architectures.
As discussed above, the increases in compute performance and the proliferation of data have helped solve two of these issues. The third problem to be solved was the model architectures.
In 2017 researchers at Google and the University of Toronto wrote the now famous “Attention is all you need” paper, introducing the transformer architecture and a new way of using the concept of attention. This was a critical breakthrough for the architectures of generative models.
Early considerations and hypotheses
At Square Peg, we segment AI into two specific categories: vertical applications and horizontal enablers.
Vertical applications are platforms that solve a specific problem for a specific set of customers. Horizontal enablers are businesses that focus on building and maintaining AI themselves.
Our hypotheses for both vertical applications and horizontal enabler businesses lead us to believe that the distribution of value across the AI landscape will form an ‘hourglass’ shape, where value is most significantly aggregated at the vertical and infrastructure layers.
Below we address our evolving set of considerations for the top two portions of the hourglass shown below, as we don’t expect to look at investing in many companies in the bottom category.
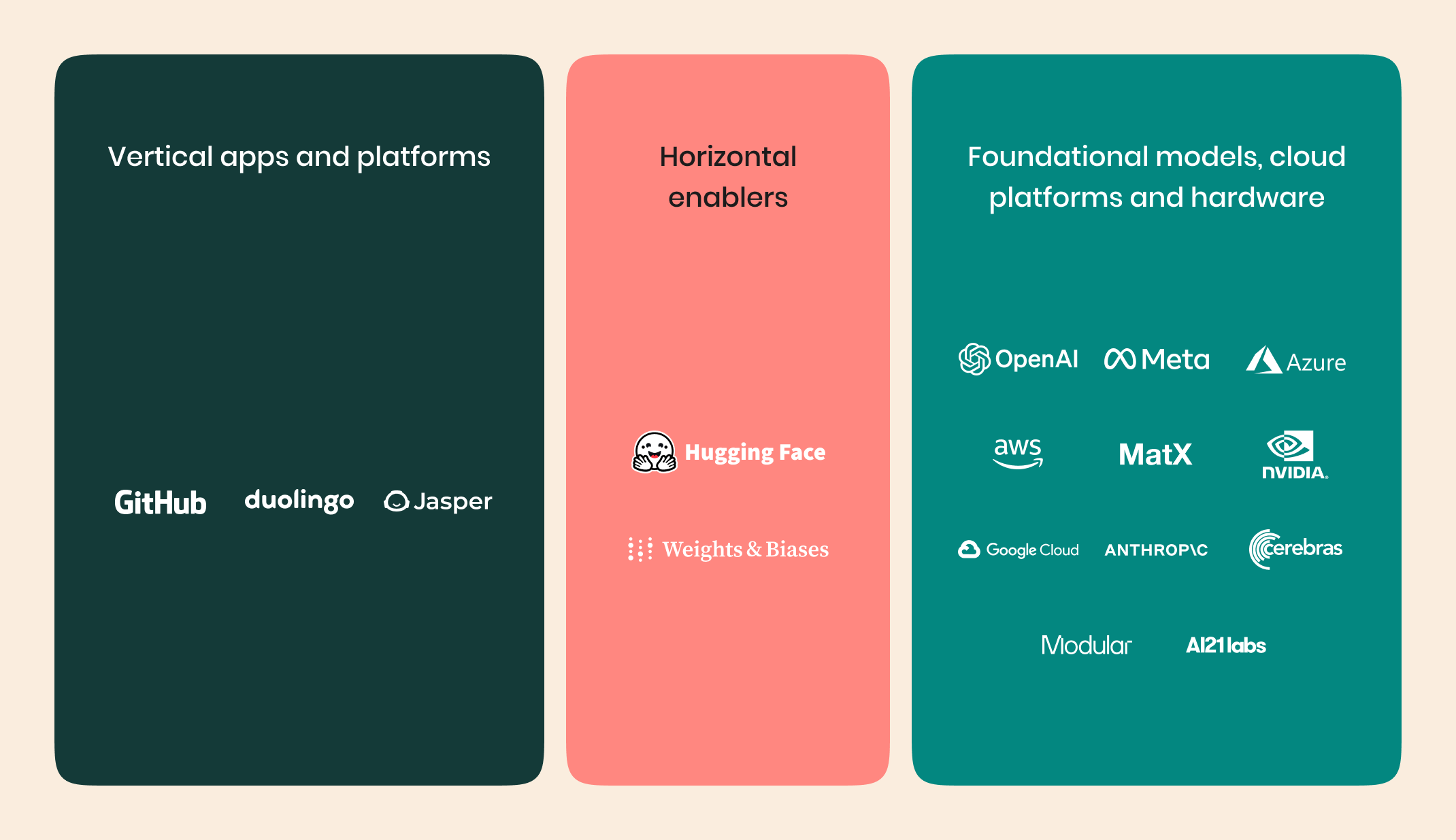
Our Hypothesis for Vertical Applications
- Broad-reaching impact = many winners. AI will be leveraged - directly or indirectly - in every industry and eventually every business. It’s not clear yet how much value will be captured by incumbents versus startups, but we do expect that there will be a large number of winners capturing value across industries.
- Data is not the moat it once was. Before we could capture the open internet’s data in a foundation model (like GPT-4), businesses needed to train their own models from scratch and that required a significant amount of data. In today’s paradigm, businesses can start using an AI model with little to no data. Additionally, because these large models are trained on much of the open internet, incremental data added to the model is less likely to be distinct from what the model was trained on and as a result does not provide a performance advantage.
- Product and GTM matter more than models. While models do matter, we don’t believe that companies will win from the marginal benefits of adopting one model over another. Great models will be a powerful tool, but companies still need to sell a product that their customers want. Founders should not lose sight of what their customers want in favour of model optimisation.
- Variable cost structures impact unit economics. Currently, most foundational models are trained using data from the open internet, and they can solve a variety of problems. However, it’s generally not cost-efficient to use the largest models for narrow use cases. While we expect to see sharp decreases in costs, we are paying more attention to how the use of AI impacts unit economics in the short term.
- Competition will be fiercer than ever. The barrier to creating a startup will be lower. Cloud significantly lowered barriers to entry for many technology startups by decreasing the upfront infrastructure investment required. AI will decrease barriers to entry again, by making coding, product development and feature delivery easier than ever.
Our Hypothesis for Horizontal Enablers
- Customer bias towards open source software. The main customer archetypes of horizontal enablers (software engineers, data engineers, data scientists and ML engineers) have a bias towards using and creating open source. Our observation is that pricing power in spaces with strong low-cost open source players is diminished and hence is something we keep in mind while assessing a business in this space.
- Customers are consistent early adopters. Engineers are early adopters of new technologies and their toolkit is always evolving, as technology itself evolves. Their willingness to try new solutions can lead to lower stickiness and hence lower retention.
- Higher risk of vertical integration. Large cloud players are aggressively focused on leveraging their existing platform advantages to move into other parts of the technology stack. Horizontal enablers hence risk being within the strategic strike zone of these players.
- More affected by technology shifts than vertical applications. This space experiences faster technological change than other spaces, meaning certain modalities or approaches can be made redundant by newer approaches. Companies, therefore, that tie themselves to a particular modality are at risk of being made redundant in the way a technology-agnostic product does not.
- Homogeneity of customer types. Because there are a smaller number of customer personas to sell than vertical applications (engineers with similar problem sets) we expect to see more of a concentrated number of players winning this opportunity.
What’s Next?
While there has been accelerated development within AI over recent years, we think that there are a few key waves still to come:
- GenAI breaks out of the lab. Today’s use cases are focused on the most obvious applications of generative AI, but we’re starting to see more novel cross-discipline applications of generative AI emerge (here’s one example in autonomous vehicles). We’re excited for this to lead to a greater number of use cases for this technology and hence a greater proliferation of exciting businesses.
- Enterprise adopts AI. Enterprises are excited about AI, but many today experience a range of issues in adopting it (like trust, safety, data engineering, interpretability and predictability to name a few). Once businesses are empowered to break through these early constraints there will be a greater pool of value for startups to capture.
- Human-level AI (AGI). How AGI is defined and when it will arrive (or if it already has) is contentious and hotly debated. What we can say, however, is that increases in the capabilities of AI models will lead to a greater volume of problems able to be solved and hence new types of businesses.

If you’re founding a business in the AI space, we’d love to hear from you. Get in touch at casey@squarepeg.vc or yonatan@squarepeg.vc.